Label Propagation in Video Sequences
Pixelwise labelled video sequences are essential for learning multi-class classifiers for video segmentation or scene recognition. However hand labelling video sequences frame-by-frame is tedious, time consuming (30 to 45 mins per frame) and it is difficult to maintain frame-to-frame label consistency.
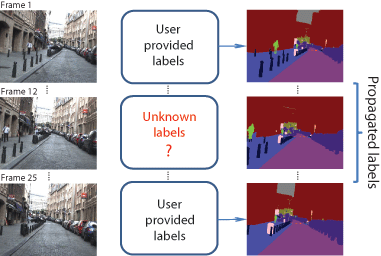
Label propagation in video sequences
We_formulate the problem as follows: given few hand-labelled frames of a video sequence, (typically the first and last frames), we aim at providing pixelwise labelling of all other frames of the video sequence along with the class probabilities.
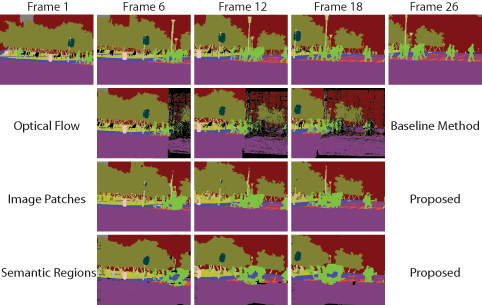
A sample result of label propagation obtained by using our probabilistic model. The proposed methods based on image patches and semantic regions are superior to a naive solution based on optical flow
Our novel probabilistic model_and inference algorithm can be based_on pixelwise correspondences obtained_with a variety of_methods. We have_compared qualitatively and quantitatively the label propagation results given by using optic flow estimates, alongside more sophisticated approaches based on image patches, as seen in epitomic models, or extraction of semantically consistent regions.
We have used the propagated labels to train a state of the art Random forest classifier for video segmentation. Compared with training on fully ground truthed data, the classification results demonstrate a minimal loss in accuracy, which supports and encourages the use of the proposed label propagation algorithm.
Project page updated on August, 1st 2014